Prize for age predictor
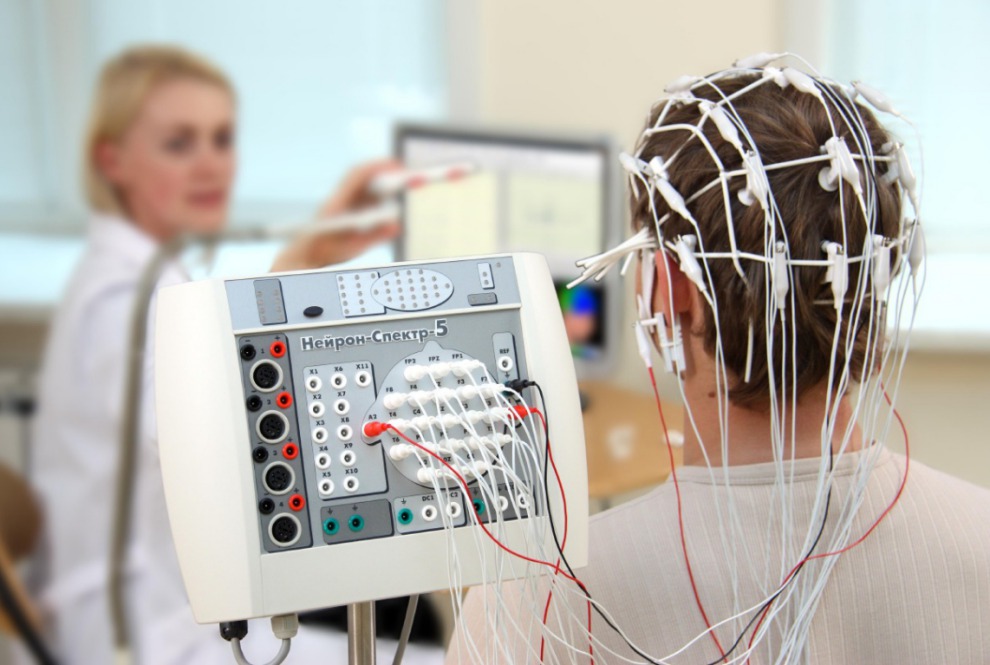
22 03 2023
Category: artificial intelligence, hackathon, prize
A team of Polish researchers, which includes Dr. Jacek Rogala from Centre for Systemic Risk Analysis, took 2nd place in a W3PHIAI-23 Aging Hackathon that challenged participants to design an optimal age predictor.
According to the organizers, the identification of biomarkers to describe, define and predict biological age is a very active topic of research. Aging affects organisms differently, and chronological age does not always coincide with biological age, a statement all the more true for diseases like progeroid syndromes and other accelerated aging conditions. Having reliable predictors of chronological age, biological age, and their relationship is important for both diagnostic and prognostic uses (e.g., for disease co-morbidity and mortality), as well as research and clinical use (e.g., rejuvenation and reprogramming, therapeutic outcome, etc.).
The Aging Hackaton, announced in January, aimed at challenging the machine learning community to design an optimal age predictor. There was no limitation or restriction on the type of data used for this challenge, and participants were encouraged to explore the use of multimodal data sources and methods to achieve the goal.
2nd Prize in the competition was won by the Polish team, consisting of Mikołaj Spytek, Weronika Hryniewska-Guzik, Jarosław Żygierewicz, Jacek Rogala, Przemyslaw Biecek. The team presented the project:
Challenges Facing the Explainability of Age Prediction Models: Case Study for Two Modalities
Description of the project:
“The prediction of age is a challenging task with various practical applications in high-impact fields like the healthcare domain or criminology.
Despite the growing number of models and their increasing performance, we still know little about how these models work. Numerous examples of failures of AI systems show that performance alone is insufficient, thus, new methods are needed to explore and explain the reasons for the model’s predictions.
In this paper, we investigate the use of Explainable Artificial Intelligence (XAI) for age prediction focusing on two specific modalities, EEG signal and lung X-rays. We share predictive models for age to facilitate further research on new techniques to explain models for these modalities.”